OBIEE11G BI Server Cache Management:-
What is Cache?
Analytics systems reduce database calls by creating a ‘Cache’ of data on the same machine as the Analytics engine. Caching has a small cost in terms of disk space to store and a small number of I/O transactions on the server, but this cost is easily outweighed by the improvement in response time.
Types of Cache in OBIEE
There are two different types of cache in Oracle Business Intelligence Enterprise Edition (OBIEE):
- The Query Cache (BI Server Cache): The OBIEE Server can save the results of a query in cache files and then reuse those results later when a similar query is requested. This type of cache is referred to as Query cache.
- The Presentation Server Cache: When users run analytics, Presentation server can cache the results
Why do we need to Purge Cache?
The main reason to implement the cache purge process is, when we have data that is being updated frequently in the warehouse and if there is a query cache present for the query that hits on the database, then the numbers may vary from that of the database. In such cases if you purge the cache the issue gets resolved.
Steps to Automatically Purge BI Server Cache
[Assuming a Oracle Business Intelligence Enterprise Edition (OBIEE) server and Data warehouse Administration Console (DAC) server are installed on the Linux machines.]
Here are the few steps to purging BI SERVER cache automatically in Oracle Business Intelligence Applications. For our purposes, the OBIEE server and DAC server are on two different Linux machines.
Step 1
As the OBIEE and DAC servers present are two different servers we need to login to OBIEE server from DAC server. This can be achieved by using the SSH command in Linux.
Step 2
As the DAC server requires password-less login, we either need to setup the RSA keys or use the ‘sshpass’ command to login to the OBIEE server. Where RSA keys are most preferable by the client personal. Setting up RSA keys is mostly the work of a DBA.
Step 3
Once the login without using a password is completed, we now need to move to the OBIEE HOME and create two files: purge.txt and purgecache.sh
We also must provide the privileges required for execution.
Thus after every ETL load the purging of the cache can be achieved.
We can check the output of the purging process by navigating to the dac/log folder and check for post_etl.sh.log file.
The Above steps help us purge the BI SERVER cache automatically after every DAC load.
Presentation Server Cache Management
The Presentation server cache can be managed in the instanceconfig.xml file and it’s a one-time setup by adding the tags shown below (just above the
Web Cache
1440 1440 1440
This one only for BI Server to Presentation server expire only. 10
A cache management strategy sounds grand doesn’t it? But it boils down to two things:
- Accuracy – Flush any data from the cache that is now stale
- Speed – Prime the cache so that as many queries get a hit on it, first time
Maintaining an Accurate Cache
Every query that is run through the BI Server, whether from a Dashboard, Answers, or more funky routes such as custom ODBC clients or JDBC, will end up in cache. It’s possible to “seed” (“prime”/“warmup”) the cache explicitly, and this is discussed later. The only time you won’t see data in the cache is if (a) you have BI Server caching disabled, or (b) you’ve disabled the Cacheable option for a physical table that is involved in providing the data for the query being run.
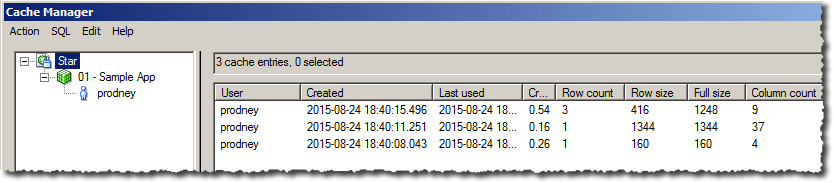
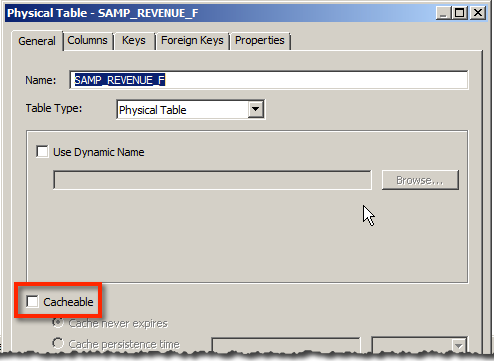
Purging Options
So we’ve a spread of queries run that hit various dimension and fact tables and created lots of cache entries. Now we’ve loaded data into our underlying database, so we need to make sure that the next time a user runs an OBIEE query that uses the new data they can see it. Otherwise we commit the cardinal sin of any analytical system and show the user incorrect data which is a Bad Thing. It may be fast, but it’s WRONG….
We can purge the whole cache, but that’s a pretty brutal approach. The cache is persisted to disk and can hold lots of data stretching back months - to blitz all of that just because one table has some new data is overkill. A more targeted approach is to purge by physical database, physical table, or even logical query. When would you use these?
- Purge entire cache - the nuclear option, but also the simplest. If your data model is small and a large proportion of the underlying physical tables may have changed data, then go for this
- Purge by Physical Database - less brutal that clearing the whole cache, if you have various data sources that are loaded at different points in the batch schedule then targeting a particular physical database makes sense.
- Purge by Physical Table - if many tables within your database have remained unchanged, whilst a large proportion of particular tables have changed (or it’s a small table) then this is a sensible option to run for each affected table
- Purge by Query - If you add a few thousand rows to a billion row fact table, purging all references to that table from the cache would be a waste. Imagine you have a table with sales by day. You load new sales figures daily, so purging the cache by query for recent data is obviously necessary, but data from previous weeks and months may well remain untouched so it makes sense to leave queries against those in the cache. The specifics of this choice are down to you and your ETL process and business rules inherent in the data (maybe there shouldn’t be old data loaded, but what happens if there is? See above re. serving wrong data to users). This option is the most complex to maintain because you risk leaving behind in the cache data that may be stale but doesn’t match the precise set of queries that you purge against.
Which one is correct depends on
- your data load and how many tables you’ve changed
- your level of reliance on the cache (can you afford low cache hit ratio until it warms up again?)
- time to reseed new content
If you are heavily dependant on the cache and have large amounts of data in it, you are probably going to need to invest time in a precise and potentially complex cache purge strategy. Conversely if you use caching as the ‘icing on the cake’ and/or it’s quick to seed new content then the simplest option is to purge the entire cache. Simple is good; OBIEE has enough moving parts without adding to its complexity unnecessarily.
Note that OBIEE itself will perform cache purges in some situations including if a dynamic repository variable used by a Business Model (e.g. in a Logical Column) gets a new value through a scheduled initialisation block.
Performing the Purge
There are several ways in which we can purge the cache. First I’ll discuss the ones that I would not recommend except for manual testing:
- Administration Tool -> Manage -> Cache -> Purge. Doing this every time your ETL runs is not a sensible idea unless you enjoy watching paint dry (or need to manually purge it as part of a deployment of a new RPD etc).
- In the Physical table, setting Cache persistence time. Why not? Because this time period starts from when the data was loaded into the cache, not when the data was loaded into your database.
An easy mistake to make would be to think that with a daily ETL run, setting the Cache persistence time to 1 day might be a good idea. It’s not, because if your ETL runs at 06:00 and someone runs a report at 05:00, there is a going to be a stale cache entry present for another 23 hours. Even if you use cache seeding, you’re still relinquishing control of the data accuracy in your cache. What happens if the ETL batch overruns or underruns?
The only scenario in which I would use this option is if I was querying directly against a transactional system and wanted to minimise the number of hits OBIEE made against it - the trade-off being users would deliberately be seeing stale data (but sometimes this is an acceptable compromise, so long as it’s made clear in the presentation of the data).
So the two viable options for cache purging are:
BI Server Cache Purge Procedures
These are often called “ODBC” Procedures but technically ODBC is just one - of several - ways that the commands can be sent to the BI Server to invoke.
As well as supporting queries for data from clients (such as Presentation Services) sent as Logical SQL, the BI Server also has its own set of procedures. Many of these are internal and mostly undocumented (Christian Berg does a great job of explaining them here, and they do creep into the documentation here and here), but there are some cache management ones that are fully supported and documented. They are:
SAPurgeCacheByQuery
SAPurgeCacheByTable
SAPurgeCacheByDatabase
SAPurgeAllCache
SAPurgeCacheBySubjectArea
(>= 11.1.1.9)SAPurgeCacheEntryByIDVector
(>= 11.1.1.9)